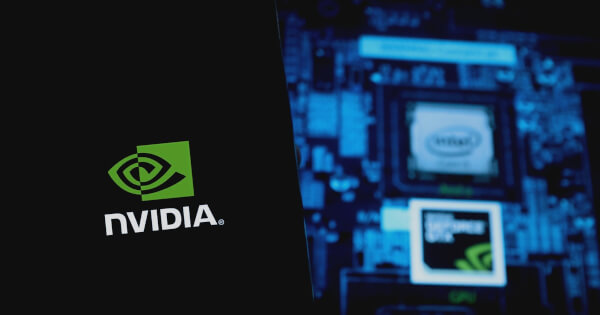
NVIDIA Enhances Data Privacy with Homomorphic Encryption for Federated XGBoost
December 19, 2024 – In a significant move to bolster the security of federated learning models, NVIDIA has integrated homomorphic encryption (HE) into its Federated XGBoost framework. This breakthrough ensures that data remains secure during computation, effectively addressing the ‘honest-but-curious’ threat model where participants may attempt to infer sensitive information.
Federated XGBoost and Its Applications
XGBoost, a widely used machine learning algorithm for tabular data modeling, has been extended by NVIDIA to support multisite collaborative training through Federated XGBoost. This plugin enables the model to operate across decentralized data sources in both horizontal and vertical settings. In vertical federated learning, parties hold different features of a dataset, while in horizontal settings, each party holds all features for a subset of the population. NVIDIA FLARE, an open-source SDK, supports this federated learning framework by managing communication challenges and ensuring seamless operation across various network conditions.
However, as XGBoost operates under an assumption of full mutual trust, NVIDIA acknowledges that in practice, participants may attempt to glean additional information from the data, necessitating enhanced security measures.
Source: Blockchain.News