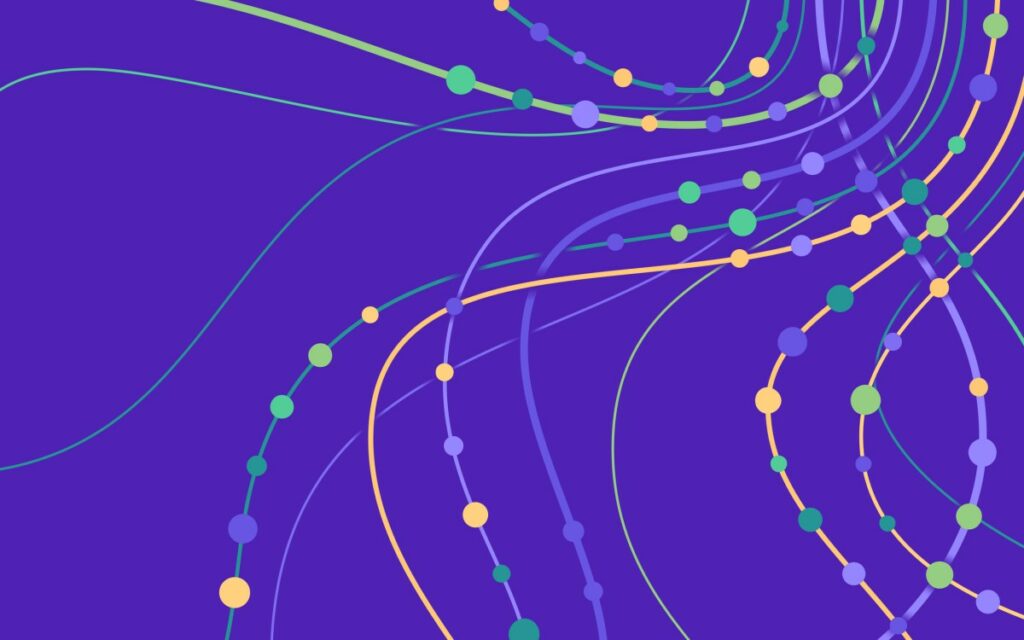
Title: A popular technique to make AI more efficient has drawbacks
Kyle Wiggers reports that a widely employed strategy to optimize artificial intelligence (AI) for reduced computational resources and memory constraints, namely quantization and low-precision arithmetic, may not be as straightforwardly beneficial as previously thought. Researchers at the University of Washington have revealed findings that suggest this approach can result in a noticeable loss of quality when applied to AI models.
As a key player in the realm of AI research, Nvidia is actively pursuing the development of lower precision for model inference, introducing its Blackwell chip with support for 4-bit precision (FP4). This move aims to address memory and power constraints faced by data centers. However, experts warn that drastically reducing the bit depth may not be advisable.
According to Kumar, a researcher involved in this study, there is an upper limit beyond which further reductions in quantization precision do not necessarily yield noticeable gains in computational efficiency. He emphasized that AI models possess inherent limitations, making it crucial to consider their unique characteristics when designing optimization strategies.
In light of these findings, the research team cautions against overly relying on lower precision as a means to reduce inference costs without sacrificing quality. In other words, there is no “free lunch” in reducing bit precision; instead, meticulous curation and filtering of high-quality data must be prioritized within smaller models.
This study serves as a valuable reminder that AI development requires nuanced understanding of the complexities involved, encouraging researchers to explore new architectures designed with low-precision training stability in mind.
Source: techcrunch.com