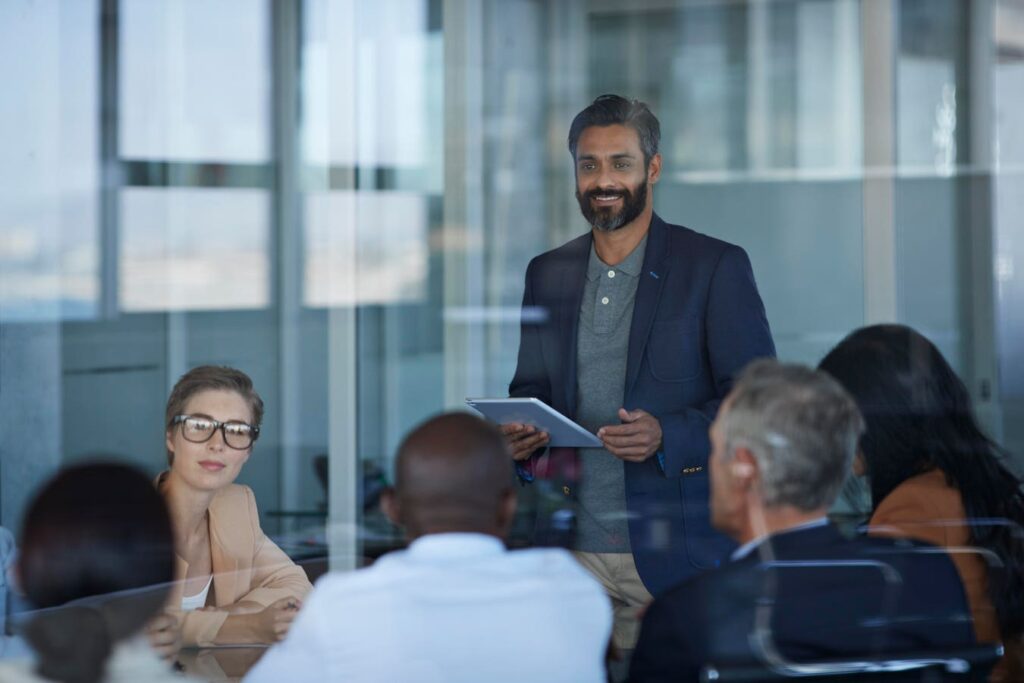
Three Challenges Stalling AI Implementation in the Financial Sector
The implementation of Artificial Intelligence (AI) in the financial sector has been touted as a revolutionary solution to transform finance and banking operations. However, despite its immense potential, AI is struggling to gain traction in the industry due to several major challenges.
Firstly, the regulatory maze poses significant hurdles for banks and other financial institutions. The deployment of AI introduces compliance issues that are becoming increasingly stringent by the year. In the European Union, for instance, organizations face heavy fines under the General Data Protection Regulation (GDPR) for mishandling consumer data, with fines reaching as high as 4% of a company’s global revenue. Moreover, the AI Act categorizes financial applications like credit scoring or fraud detection as “high-risk,” necessitating additional disclosures, auditing, and explainability – all of which add to compliance and administration costs.
In addition to these regulatory challenges, integrating AI with legacy systems is proving costly and complicated. The majority of banks, insurance companies, and asset management organizations have significant investments in outdated infrastructure, often dating back decades. As a result, these institutions rely on mainframe-based operations that are not structured for the heavy computation loads or complex data analytics required by AI. To overcome this issue, banks need to invest extensively in bespoke repairs to update their systems – an expense that many smaller financial institutions cannot afford.
This technological divide is only exacerbated by the difficulties of integrating AI with legacy systems, which often use incompatible data formats. This limits the effectiveness of AI algorithms, preventing them from accessing siloed customer data across various departments within an organization. The lack of interoperability results in fragmented AI systems that cannot tap into the complete dataset required for their accuracy. Ultimately, this prevents AI from delivering on its promise of personalized customer experiences and risk analytics.
Lastly, creating customer-ready solutions with AI is proving a daunting task. Financial firms are struggling to develop usable, scalable, and trustworthy AI-powered services that can win over consumers. For instance, AI-powered customer-service bots have been met with criticism due to their limited ability to resolve problems and poor hand-offs with human agents. Moreover, crafting AI-driven solutions that deliver seamless customer experiences reliably is an uphill battle.
Furthermore, a lack of transparency surrounding AI applications is another significant concern. In industries where decisions can significantly impact financial outcomes – such as credit assessment and investment advice – opaque decision-making processes could cloud any conclusion. The drive towards explainable AI (XAI) aims to provide clear and simple explanations for complex computations, thereby enhancing trust with customers.
Lastly, the industry is grappling with a talent shortage, as 80% of community banks and credit unions list staffing as their biggest concern. Even institutions with moderate budgets are finding it challenging to recruit and retain talent capable of constructing AI solutions from scratch. This scarcity of skilled professionals hinders the widespread adoption of AI in finance.
In conclusion, while AI has immense potential to revolutionize financial services, its implementation is being hindered by numerous structural and strategic challenges. Until these hurdles are addressed, AI will remain an elite tool accessible only to a select few institutions capable of meeting the financial, regulatory, and operational demands.
Source: www.forbes.com