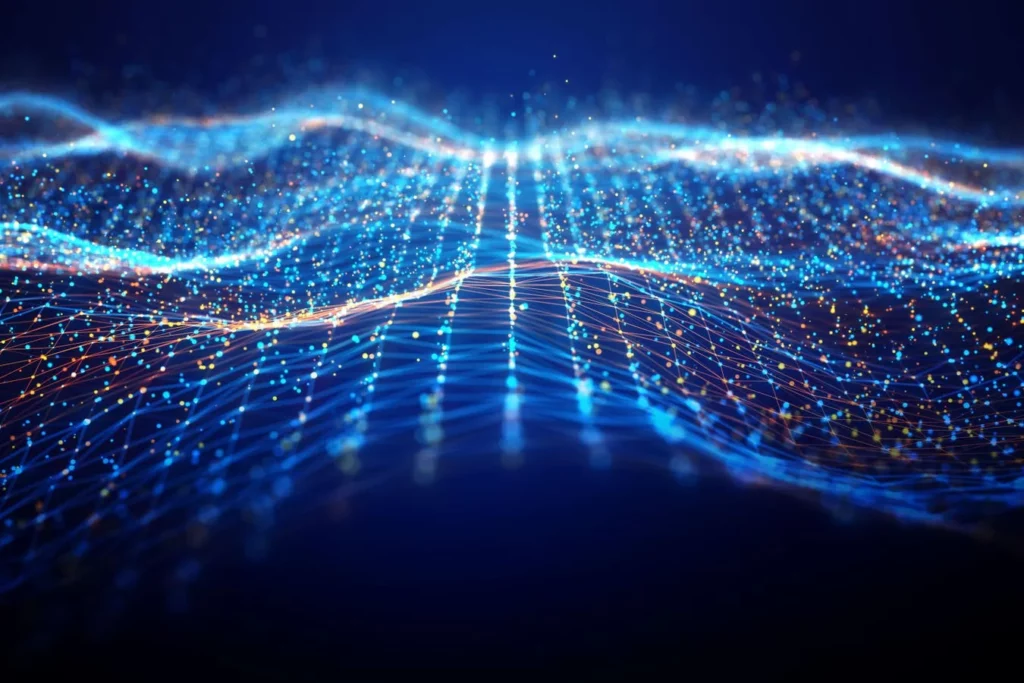
Title: Data Governance: Ensuring Trust, Compliance and Quality at Scale
As organizations rely increasingly on artificial intelligence (AI) to drive decision-making, manage risk, and fuel innovation, the importance of robust data governance cannot be overstated. In this article, we’ll explore how AI-driven tools can revolutionize data governance, enabling trust, compliance, and quality at scale.
The Future of Data Governance: Transparency and Trust
In today’s digital landscape, data is no longer just a byproduct of business operations; it has become the lifeblood of organizations. However, as reliance on AI grows, so does the risk of operational inefficiencies, financial penalties, and data breaches that can cost millions. It’s crucial to recognize that data governance isn’t just about compliance – it’s about trust.
Companies must not only manage their data but also ensure it drives better decisions, fosters innovation, and ensures long-term success. The organizations that take action today will lead the industry in building scalable, transparent, and resilient data ecosystems for the future.
AI-Driven Data Governance: A New Era of Oversight
The rise of AI has transformed the way we approach data governance, enabling us to move from detection to resolution – systems that not only identify issues but resolve them automatically. These self-healing ecosystems can monitor data pipelines, detect inconsistencies, and apply fixes in real-time without manual intervention.
This shift is already underway in high-frequency industries like trading and e-commerce, where speed and precision are everything. Whether it’s detecting an API failure, fixing a broken pipeline, or correcting a data mismatch, these intelligent systems are transforming how we manage and govern enterprise data.
Four Essential Strategies for Successful Adoption
As leaders ready to implement AI-powered governance, the focus has shifted from “why” to “how.” Success depends not just on technology but on execution. To ensure seamless adoption, consider the following essential strategies:
1. Start small, prove value, then scale: Begin with a targeted, high-impact use case – like data reconciliation or anomaly detection. Demonstrate clear value quickly and build momentum by expanding to broader initiatives.
2. Engage stakeholders and build for transparency: Involve cross-functional teams early, including IT, finance, compliance, and legal. Prioritize platforms that support explainability, traceability, and auditability to meet regulatory and organizational accountability needs.
3. Embed governance into everyday workflows: Ensure AI tools are integrated with existing data processes, supported by rich metadata and documentation. Use human-in-the-loop designs for decisions that carry risk, so AI augments – rather than replaces – critical judgment.
4. Measure, adapt, and support change: Define success metrics from day one (e.g., data accuracy, compliance efficiency, time saved). Track performance, iterate on results, and invest in training to support long-term adoption and cultural change.
Conclusion
Data governance is no longer a choice; it’s an imperative for any organization seeking to maintain trust with stakeholders, regulators, and the public. As AI continues to revolutionize data management, leaders must recognize that transparency, accountability, and human oversight are essential components of responsible AI development. By adopting these strategies, organizations can harness the power of AI-driven governance to drive innovation, optimize risk management, and build a future where trust is the foundation upon which every decision is made.
About the Author
[Your Name] is a [Your Title] at [Company].
Source: https://www.forbes.com/councils/forbestechcouncil/2025/04/30/data-governance-ensuring-trust-compliance-and-quality-at-scale/