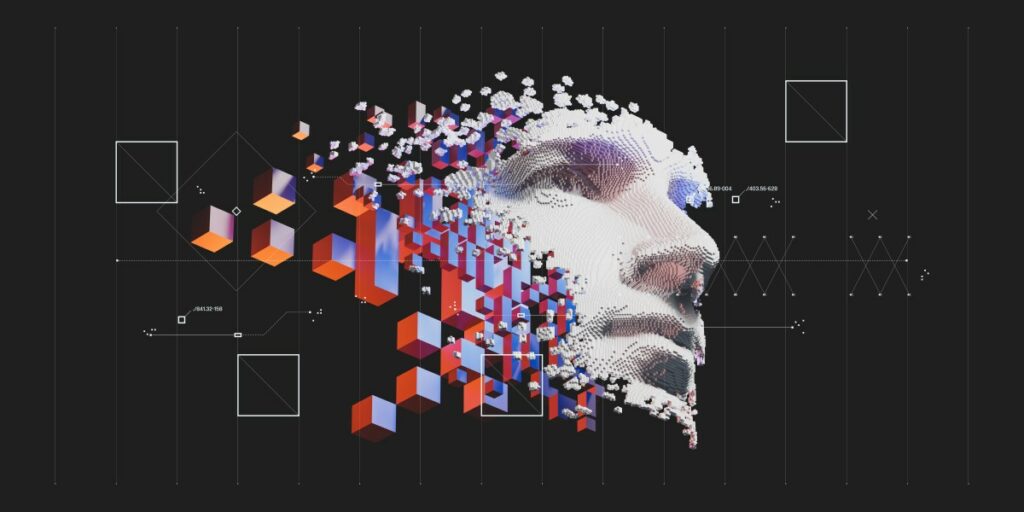
The Promise and Perils of Synthetic Data
In recent years, the use of synthetic data in AI model training has become increasingly popular. This approach involves generating artificial data sets to augment or replace real-world data, which is often limited, biased, or expensive to collect. However, a new study suggests that relying solely on synthetic data could have unforeseen consequences.
Researchers at Stanford University and the University of California found that models trained exclusively on synthetic data are prone to forget their training data over generations, leading to reduced performance and less diverse responses. In other words, these models become “forgetful” and lose their ability to provide innovative or even accurate answers.
The study, which focused on language models, revealed that the more a model relies on synthetic data, the more it becomes biased towards generic or irrelevant answers. This phenomenon is particularly concerning when considering the potential implications of AI-driven chatbots, image generators, and other applications that rely heavily on these models.
“We need to examine the generated data, iterate on the generation process, and identify safeguards to remove low-quality data points,” warned Soldaini, a researcher at Stanford University. “Synthetic data pipelines are not a self-improving machine; their output must be carefully inspected and improved before being used for training.”
The findings have significant implications for AI researchers, who may need to reconsider the role of synthetic data in their research and development processes. While generating large amounts of high-quality synthetic data is challenging, it’s clear that relying solely on this approach could lead to catastrophic consequences.
Moreover, the study highlights the urgent need for more robust quality control measures to ensure that any generated data meets specific criteria before being used to train AI models. It’s essential to recognize that AI models are only as good as the data they’re trained on and that synthetic data should be used in conjunction with real-world data whenever possible.
The results of this study also underscore the importance of transparency and accountability in AI development. As AI systems become increasingly prevalent, it is crucial for researchers and developers to be transparent about their methods and to prioritize fairness, accuracy, and relevance in AI-driven applications.
In conclusion, while synthetic data may offer a promising solution for improving AI performance and efficiency, it is essential to approach this technology with caution and rigorous quality control measures.
Source: techcrunch.com