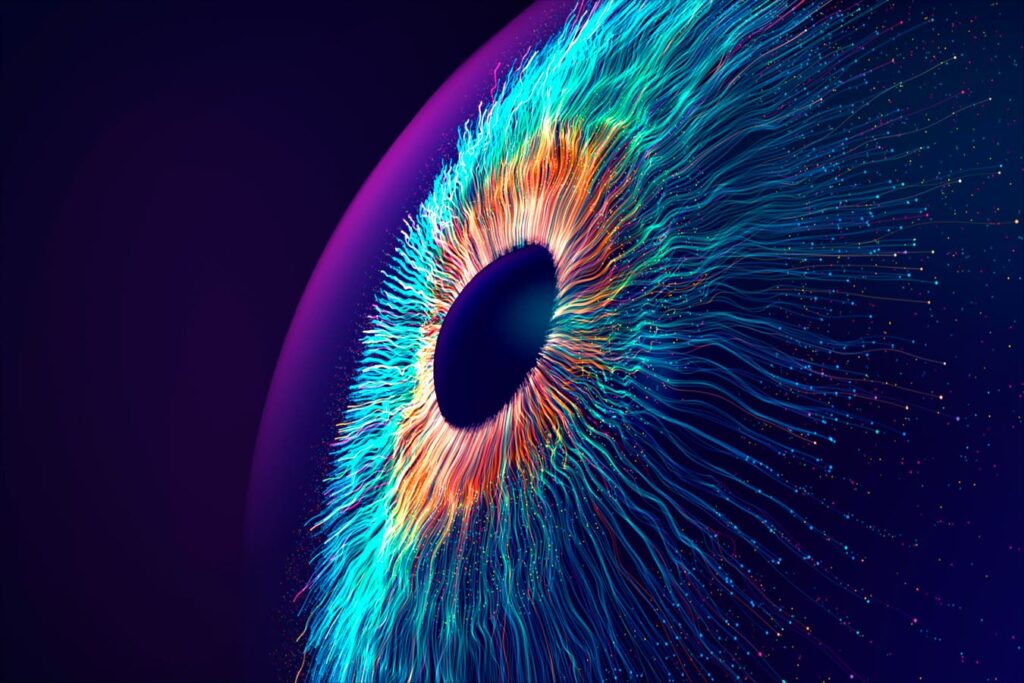
AI And ML In Observability: Hype Or Helpful?
The landscape of artificial intelligence and machine learning (AI/ML) advancements has significantly impacted the field of observability. As we venture into uncharted territory, one question persists: How will AI/ML fundamentally change our approach to observability? While some assert that AI/ML could replace engineers and SREs entirely, reality is starkly different.
In today’s complex systems, traditional analysis methods are no longer sufficient. The need for more critical thinking in the form of connecting dots and making inferences becomes increasingly necessary. For instance, consider a massive e-commerce platform processing millions of transactions daily. While metrics such as CPU data, network latency, and query times provide valuable insights into individual component performance, they fall short of capturing the entire system’s behavior.
To grasp the overall health and dynamics, observability teams must consider the broader context and relationships. This involves considering factors like peak shopping seasons, promotional campaigns’ effects on inventory and logistics, and so forth. In this dynamic environment, the system architecture becomes “unknowable” in the traditional sense – any attempt to capture it is instantly outdated.
The observability team can no longer rely solely on static models or pre-defined thresholds to identify and resolve issues. Instead, they must adopt a more iterative approach, where they uncover system behavior through telemetry, real-time action, and feedback loops. Techniques like distributed tracing for end-to-end transaction flow analysis, real-time anomaly detection for emerging trends and patterns recognition, and incident response to quickly diagnose and mitigate issues are essential in this environment.
AI/ML will undoubtedly assist in some aspects of these processes but human input, understanding, and contextualization remain essential. This is particularly true during the early days of generative AI/ML, where LLMs (Large Language Models) come with biases, hallucinations, and other bugs. These issues will be resolved over time, but it remains unclear whether AI/ML will ever develop the deep understanding and contextual awareness necessary for effective observability.
However, there are opportunities for AI/ML to make a meaningful impact in observability. Initially, focus has been on minimizing toil and automating specific tasks like anomaly detection and root cause analysis, freeing engineers to tackle more complex issues. For example, AI/ML models can be trained on historical monitoring data to learn normal system behavior patterns, enabling accurate anomaly detection and alerting engineers before problems escalate.
The real efficiency gains will come from AI/ML augmenting developers’ experiences. Junior site reliability engineers (SREs) will gain access to the knowledge of an experienced expert at their fingertips. Moreover, they won’t have to spend years learning programming languages like PromQL or SQL; instead, they can use natural language interfaces to generate queries and interact with observability systems.
An SRE could simply type “Show me CPU utilization on our web servers over the past week,” and AI/ML would translate that into an appropriate query. More sophisticated use cases involve self-optimizing usage automatically. For instance, LLMs can analyze profiles and recommend changes to conserve CPU and memory – saving considerable time and resources.
This opens up the possibility for non-technical roles such as product managers, executives, and business analysts to leverage observability data directly by querying and analyzing system behavior, performance, and reliability using conversational AI/ML assistants.
Source: www.forbes.com