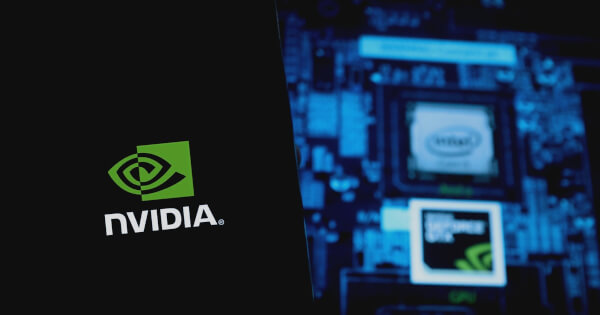
GenMol: A New Frontier in Molecular Generation for Drug Discovery
In the rapidly evolving field of computational drug discovery, a new model named GenMol is revolutionizing the game by offering a versatile approach to molecular generation. As per NVIDIA’s blog, GenMol is poised to redefine how researchers tackle drug discovery tasks with its innovative framework.
Traditional drug discovery models often require significant adaptation to address new tasks, demanding extensive time, computational resources, and expertise. GenMol, on the other hand, provides a generalist framework capable of handling diverse drug discovery tasks by leveraging a chemically intuitive setup. This model aims to simplify the drug discovery process by enabling dynamic exploration and optimization of molecular structures.
GenMol is compared with SAFE-GPT, a previous model known for its sequential attachment-based fragment embedding (SAFE) representation. While SAFE-GPT was a significant advancement at its time, GenMol addresses its limitations in efficiency and scalability. GenMol’s discrete diffusion-based architecture and parallel decoding offer enhanced computational efficiency and broader task versatility, outperforming SAFE-GPT in various drug discovery tasks.
The molecular representation is crucial for the accuracy and flexibility of computational models. GenMol uses the SAFE representation, breaking down molecules into modular fragments, unlike traditional linear notations like SMILES. This method facilitates scaffold decoration, motif extension, and other complex tasks, offering a more intuitive approach to molecular design.
GenMol’s architecture allows for parallel, non-autoregressive decoding with bidirectional attention, enabling simultaneous processing of molecular fragments. This capability enables GenMol to outperform SAFE-GPT in fragment-constrained molecule generation tasks, achieving higher quality scores in motif extension, scaffold decoration, and superstructure generation.
Moreover, GenMol’s discrete diffusion framework significantly enhances generation efficiency, offering up to 35% faster sampling compared to SAFE-GPT. This makes GenMol highly scalable for industrial-scale drug discovery, reducing computational overhead in large-scale or high-throughput scenarios.
In conclusion, GenMol represents a critical advancement in AI-driven drug discovery, providing a versatile, efficient, and precise tool for researchers. Its ability to handle diverse tasks without the need for task-specific adaptation marks a significant leap forward in molecular generation.
Source: Blockchain.News