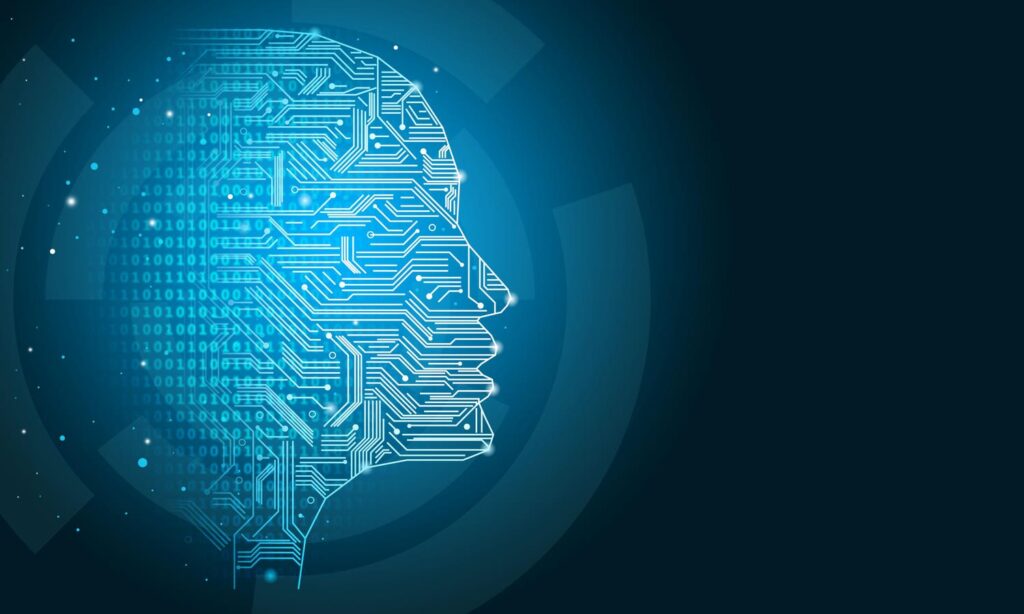
The Enterprise AI Revolution: Opportunities And Obstacles In 2025
As we step into the new year of 2025, the enterprise landscape is undergoing a profound transformation. Artificial intelligence (AI) has become an indispensable tool for organizations seeking to optimize their processes, enhance customer experiences and drive innovation. However, embracing AI also presents numerous challenges that must be addressed.
On one hand, AI offers immense opportunities across various sectors. Companies are leveraging generative AI to revolutionize customer interactions, refine enterprise search capabilities, and even automate financial analysis and reporting tasks. The technology sector has already adopted AI-assisted development tools, which have transformed the way developers write code, debug applications, and automate testing.
Moreover, personalization at scale is now possible thanks to AI, enabling businesses to deliver tailored experiences to customers. For instance, Duolingo uses generative AI to create customized language exercises tailored to individual learning patterns. This level of customization extends across industries, including e-commerce product recommendations and financial service offerings.
Despite the plethora of opportunities, numerous obstacles stand in the way of widespread AI adoption. Firstly, maintaining fresh, accurate data in AI systems poses a significant challenge. It is akin to attempting to hit a moving target while standing on shifting sand. Organizations must invest in mechanisms for continuous data ingestion and synchronization, detection of data drift and model performance degradation, version dependencies between data sources and models, and efficient updating of models without complete retraining.
Secondly, security remains a paramount concern. Enterprises must protect sensitive data, comply with access control requirements, prevent unauthorized data exposure, and implement effective guardrails against misuse. Moreover, the increased risk of derivative risks from AI-generated content necessitates careful management.
The need for flexible architectures that can support multiple models with varying resource requirements, enable easy switching between different AI models, handle increased load during peak times, maintain acceptable latency for real-time applications, and integrate seamlessly with existing systems also presents an obstacle. This requires organizations to strike a delicate balance between the costs of AI implementation, including the high expenses of commercial LLM APIs, infrastructure costs for model deployment and scaling, hidden costs in testing and iteration, and training and maintenance expenses.
Lastly, ensuring quality assurance testing becomes a critical challenge as AI systems require long validation cycles requiring human evaluation, comprehensive regression testing with each update, gathering and incorporating user feedback, and maintaining consistency across different environments.
To successfully navigate these challenges, I recommend the following strategic approaches:
1. Embracing A Hybrid Strategy: Implement a mix of domain-specific and enterprise-wide AI solutions to allow for specialized functionality while maintaining centralized oversight.
2. Investing In Platform Architecture: Develop flexible, modular platforms that can accommodate rapid technological changes and support multiple use cases across the organization.
3. Implementing Strong Governance: Establish comprehensive data governance and security frameworks before deploying AI solutions at scale.
4. Focusing On Cost Optimization: Consider using smaller, specialized models for rudimentary tasks while reserving larger models for complex operations that require their capabilities.
5. Building Robust Testing Frameworks: Develop automated testing systems while maintaining human oversight for critical evaluations.
In conclusion, the future of enterprise AI depends on the ability to balance innovation with practical considerations of security, cost, and maintainability. Organizations must remain adaptable, implementing flexible architectures that can evolve with technological advances while maintaining strong security and governance frameworks.
Source: www.forbes.com